Why leveraging artificial intelligence in legal tech is a challenge?
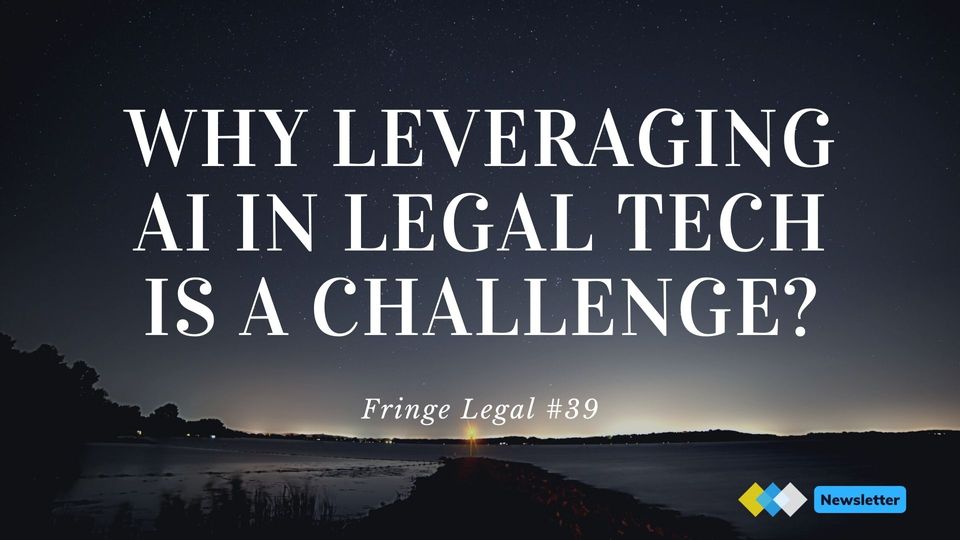
Products powered by artificial intelligence work best when used on familiar datasets, i.e., the types similar to those used during the training phase. This is generally a challenge but becomes an interesting problem to solve when dealing with legal matters.
(listen to the full episode here)
In the latest episode of the Fringe Legal podcast, I speak with Matthew Golab. Matthew is the Director of Legal Informatics and R+D at Gilbert + Tobin.
Looking at problem-solving in legal when it comes to AI, there are some unique challenges. I’m excited about this episode because we explore some of those challenges today, and even though we dive straight in, we make sure to explain as much of the jargon while we speak.
There are two points that we touched on during our chat that I wanted to explore further.
General-purpose systems vs. specialist systems
One of the biggest challenges in creating any type of AI model for a legal use case is that you are working within a closed system. Because so much of the material is sensitive, privileged, or confidential, it makes it a challenge to feed large amounts of data or high variability of information.
I thought about some of the similarities between AI strategy between the US and China - and coincidentally, Rob May, a Partner at PJC who invests in AI and robotics, covered this topic recently in his newsletter:
Since one problem with ML/AI models is getting labeled data, in a market that is more open, we will see more entrepreneurs try to obtain labeled data sets that may not be obviously valuable. In fact, one of the things VCs look for is entrepreneurs who realize a "secret." We want to back founders who understand the the entire world is mostly missing something that they understand and can use to capitalize into a business.
Many of these "secrets" will turn out to be wrong, but the few that are correct will lead to huge companies. And in a market where an entrepreneur can pursue a path of getting some labeled data that may not make sense initially to anyone else, might give that company, and the country it's based in, a boost down the road.
On the flip side, state directed capitalism might allow data sets to be captured that aren't allowed in other more democratic countries. That means use cases for ML can emerge in places like China that can't emerge in the U.S. Looking at this through a lens of probabilities around outcomes, I don't know how to handicap it more towards one country so I'd say it's a draw.
He proposes that levering “small data AI is possible by using synthetic data to change small data sets into larger ones.”
MIT News has one of the most memorable definitions of synthetic data:
Synthetic data is a bit like diet soda. To be effective, it has to resemble the “real thing” in certain ways. Diet soda should look, taste, and fizz like regular soda. Similarly, a synthetic dataset must have the same mathematical and statistical properties as the real-world dataset it's standing in for. “It looks like it, and has formatting like it,” says Kalyan Veeramachaneni, principal investigator of the Data to AI (DAI) Lab and a principal research scientist in MIT’s Laboratory for Information and Decision Systems. If it's run through a model, or used to build or test an application, it performs like that real-world data would.
I wonder if synthetic data sets are available for the legal profession today or might be soon? Maybe one of the large tech players with huge labeled datasets (such as precedent banks) could offer synthetic data set as a service that could be leveraged to build more accurate models.
Want to read more on this topic, here’s why small data is the future of AI.
Balancing high-risk tolerance against continuous improvement
During the episode, I shared a general overview of the development process used to train artificial intelligence models. A critical part of the process comes post-deployment: monitoring/observation and course correction.
Matthew made a great observation whereby with a technologists hat on, one's risk tolerance is much higher than the end-user:
To you, as a technologist, your tolerance for is it good enough is maybe say at 80%, or you're just very happy it actually didn't fail. But to a lawyer, they just assume if it's going to augment their effort or multiply their efforts, that it will be at least as good as them.
This creates a hideous loop, especially when it comes to machine learning. Once the model is created, it needs to be tested on realistic scenarios (and adjusted accordingly), but if your risk tolerance is so high as not to allow for use on "real" documents, you end up between a rock and a hard place.
You really don't have any margin of error for monitoring and correction. And that means that you're stuck between a rock and a hard place where you continuously test on closed datasets and therefore end up creating something that works well only on certain types of documents, but not well on “real” documents that you need it to work on.
It’s not just a challenge of risk management, but of time as well. If one had infinite time to work using both the current method and in parallel, experient using new technology (with the right safeguards), folks would jump at the chance.
Where I have seen this work is through the use of specialist teams that essentially do the parallel work - then it becomes a question of resource management (at least in the short term).
Show notes
- Introduction to the podcast (0:28)
- The deliberate use of language in legal vs. general language training sets (4:51)
- NLP, NLG, and NLU (7:08)
- Overview of the AI development process (9:49)
- The deliberate balance to be achieved when introducing technology to lawyers (13:22)
- High-risk tolerance as a barrier to continuous learning (15:09)
- The general sentiment about AI and the future (17:08)
- The challenge of working with different jurisdictions and languages (22:34)
- Training a specialist system vs a general-purpose system (26:37)
- The biggest improvement in tech within law firms (29:17)
You can listen to the full episode here, or wherever you listen to podcasts.
How did you like this article?
Become a Fringe Legal member
Sign in or become a Fringe Legal member to read and leave comments.
Just enter your email below to get a log in link.